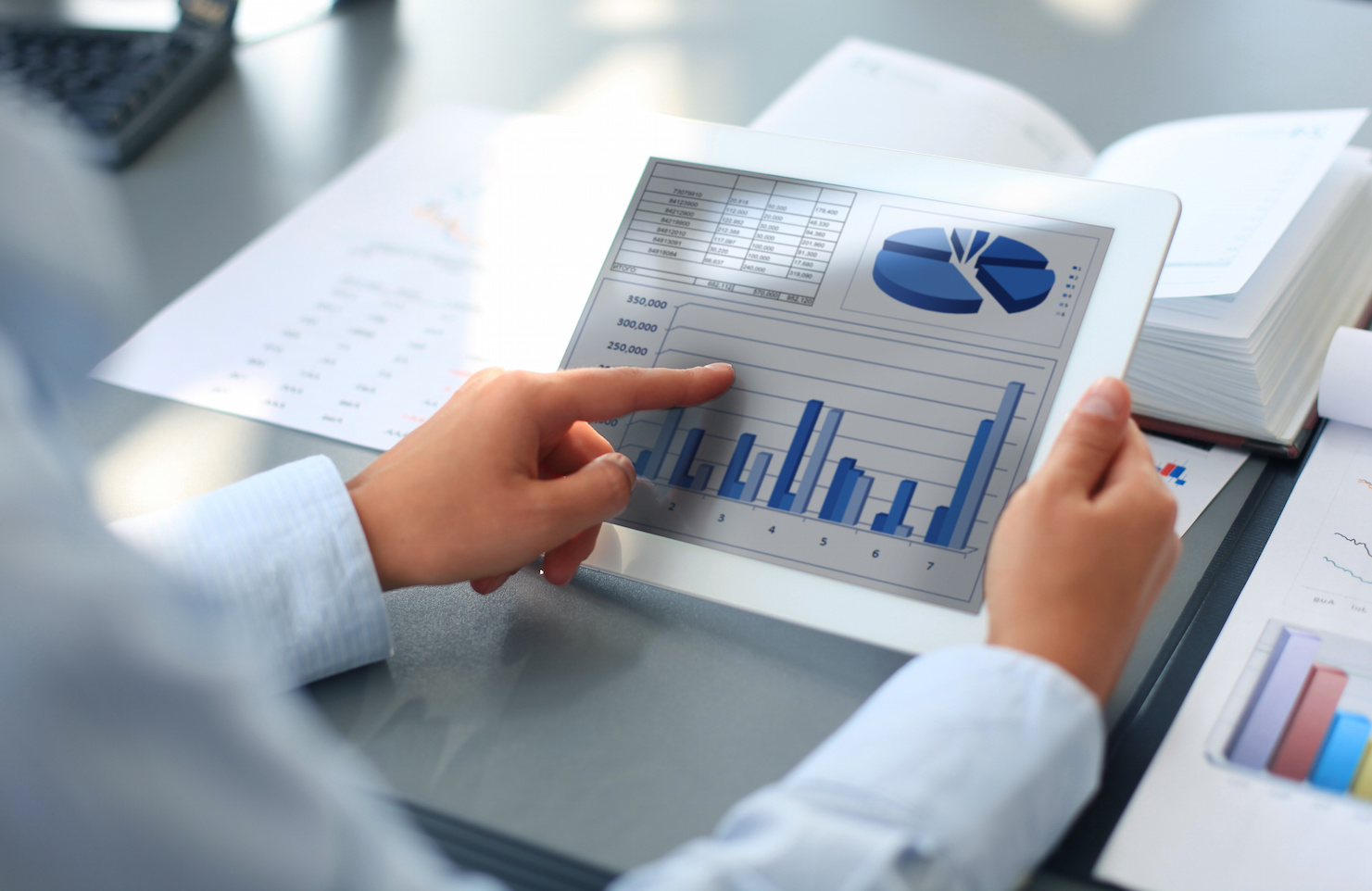
In the age of information, data has become an invaluable asset, shaping the way we live, work, and make decisions. Data-Driven Decision Making(DDDM) is a methodology that leverages the power of data to guide choices and actions. It's a paradigm shift from relying on intuition and experience to embracing empirical evidence and analysis.
In this era of unprecedented data availability, DDDM has emerged as a transformative force across industries, offering precision, efficiency, and competitive advantage like never before. Let's delve into the world of Data-Driven Decision Making and understand why it's more than just a buzzword - it's a fundamental shift in how we navigate the complexities of our data-rich world.
What Is Data-Driven Decision Making (DDDM)?
Data-driven decision-making (DDDM) is the process of making businesschoices based on facts, metrics, and data that are in line with your goals, objectives, and initiatives. When organizations understand the full value of their data, everyone, whether they are a businessanalyst, sales manager, or human resources specialist, is able to use data every day to make better choices. But this can't be done by just picking the right analytics technology to find the next strategic change.
Your company needs to make data-driven decisions the norm, which means building a culture that encourages people to think critically and be curious. People at all levels have talks that start with data, and they get better at using data by doing it.
At its core, this needs a self-service model that lets people get to the info they need while keeping security and governance in mind. It also needs to be done well, which gives workers chances to learn data skills through training and development. Lastly, having support from the top and a group that makes decisions based on data will encourage other people to do the same.
Setting up these core skills will make it easier for people at all job levels to make decisions based on data, so business groups will regularly question and look into information to find powerful insights that drive action.
Importance Of Data-Driven Decision Making
The importance of data-driven decision-making (DDDM) cannot be overstated in today's data-driven world. Here are several key reasons why DDDM is crucial:
- Precision and Accuracy -DDDM enables decision-makers to base their choices on concrete evidence rather than intuition or anecdotal information. This leads to more precise and accurate decision-making.
- Risk Reduction - Making decisions without data can be risky. DDDM allows organizations to anticipate potential issues, assess their impact, and take proactive measures to mitigate risks.
- Efficiency and Cost Savings -Data analysis often reveals inefficiencies and areas where resources can be optimized. Implementing data-driven strategies can lead to significant cost savings and operational efficiencies.
- Competitive Advantage -In highly competitive industries, organizations that harness data effectively can identify market opportunities, respond to customer needs faster, and stay ahead of their competitors.
- Innovation -Data can uncover new insights and opportunities that might not be apparent through traditional decision-making methods. DDDM fosters innovation by encouraging exploration and experimentation.
- Customer Satisfaction -Understanding customer preferences and behavior through data analysis can lead to improved products, services, and customer experiences, ultimately enhancing customer satisfaction and loyalty.
- Real-time Decision-Making -In today's fast-paced business environment, timely decisions are essential. Data-driven insights can be generated in real time, allowing organizations to respond quickly to changing conditions.
- Strategic Planning -DDDM provides a solid foundation for strategic planning. Organizations can align their long-term goals and objectives with data-driven insights, increasing the likelihood of success.
- Continuous Improvement -Data-driven organizations are more inclined to engage in a cycle of continuous improvement. They use data to evaluate the outcomes of their decisions and make adjustments as needed, leading to ongoing enhancements.
- Transparency and Accountability -DDDM encourages transparency in decision-making processes. With data as the foundation, it becomes easier to explain and justify decisions to stakeholders, fostering accountability.
- Personalization -In fields like marketing and e-commerce, data-driven approaches enable the personalization of content, recommendations, and offerings, which can significantly enhance customer engagement.
- Predictive Capabilities -Data-driven models and analytics can be used for predictive purposes, such as forecasting future trends, demand, or customer behavior, allowing organizations to plan and adapt accordingly.
Data-driven decision-making is no longer a luxury; it's a necessity for individuals, businesses, and organizations looking to thrive in an increasingly complex and data-rich environment. By leveraging data to inform choices, organizations can make more accurate, efficient, and strategic decisions, ultimately driving success and innovation in their respective domains.
Key Factors When Making Data-Driven Decisions
When making data-driven decisions, several key factors should be considered to ensure the effectiveness and reliability of the decision-making process. Here are some essential factors to keep in mind:
- Data Quality - The quality of data used for decision-making is paramount. Ensure that the data is accurate, complete, and up-to-date. Data should also be free from errors, inconsistencies, and biases.
- Relevance - Focus on collecting and analyzing data that is directly relevant to the decision at hand. Avoid the temptation to include excessive or irrelevant data, which can lead to confusion and inefficiency.
- Data Sources - Consider the sources of your data. Are they reputable and trustworthy? Evaluate the credibility and reliability of data sources to reduce the risk of using unreliable information.
- Data Governance - Establish clear data governance policies and procedures. Define data ownership, data access rights, and data security protocols to ensure that data is handled appropriately and securely.
- Data Privacy and Compliance - Adhere to data privacy regulations and ethical guidelines when collecting and using data. Protect sensitive and personally identifiable information to maintain trust and compliance.
- Analysis Techniques - Select appropriate data analysis techniques and tools based on the nature of the data and the objectives of the decision. Use statistical methods, machine learning, or other analytical approaches as needed.
- Expertise and Skills - Ensure that the individuals involved in the decision-making process have the necessary data literacy and analytical skills. Provide training if needed to bridge knowledge gaps.
- Clear Objectives - Define clear and specific objectives for the decision. What problem are you trying to solve, and what outcomes are you aiming for? Clear objectives help guide the data analysis process.
- Hypothesis Formulation - Develop hypotheses or questions to be addressed through data analysis. Having a clear hypothesis ensures that the analysis remains focused and purposeful.
- Contextual Understanding - Consider the broader context surrounding the decision. How does the decision fit into the organization's goals and strategy? Understanding the context helps in making informed choices.
- Stakeholder Involvement - Engage relevant stakeholders in the decision-making process. Different perspectives and expertise can contribute to a more well-rounded understanding of the data and its implications.
- Risk Assessment - Identify and assess potential risks associated with different decision options. Consider both the risks of taking action and the risks of inaction.
- Data Visualization - Use data visualization techniques to communicate insights effectively. Visual representations of data can make complex information more understandable for decision-makers.
- Testing and Validation - If applicable, conduct tests or experiments to validate data-driven insights and hypotheses. This helps in verifying the reliability of the data analysis.
- Documentation and Transparency - Document the entire data-driven decision-making process, including data sources, analysis methods, and the rationale behind the final decision. Transparency builds trust and accountability.
- Continuous Improvement - Embrace a culture of continuous improvement in data-driven decision-making. Learn from past decisions, gather feedback, and adapt your processes to enhance future outcomes.
Steps In Implementing Data-Driven Decision Making
It takes practice to make choices based on data. If you want to get better at being a leader, you need to know how toturn raw data into steps that can be taken to move your company forward. When you look at data, the steps below can help you make better choices.
Know Your Vision
You need to know what your company's plans are for the future before you can make good choices. This lets you make choices based on both facts and plans. Graphs and numbers don't mean much without a story to back them up.
Tip -Your company's annual objectives and key results (OKRs) or quarterly team key performance indicators (KPIs) can help you make choices based on data.
Find Data Sources
Once you know what you want to achieve, you can start gathering information. Depending on what kind of info you're collecting, you'll use different tools and sources. Use a general reporting tool if you want to look at sets of data about how your company works internally. Reporting tools give you a single place to look to see how work is going across your whole company.
Some reporting tools, like Microsoft's Power BI, let you get information from many different places outside of the program. You can use one of these tools to look at marketing trendsor how you compare to your competitors. Some general measures of success you might want to look at are:
- Gross profit margin -The gross profit margin is the difference between the company's net sales and the cost of things sold.
- Return on investment (ROI)- Return on investment (ROI) is the ratio between the income and the amount invested. ROI is often used to decide if putting time or moneyinto a project is worth it. As a business metric, it is usually used to track how well a project is doing.
- Productivity- Productivity is a way to measure how well your business makes goods or provides services. Divide the total result by the total input to figure this out.
- Total number of customers- This is an easy but useful way to measure progress. The more people who paid, the more moneythe business made.
- Recurring revenue- This is the amount of money made by all of your active users during a certain time period. It is often used by SaaS companies. It's usually measured either once a month or once a year.
Depending on your job and your goals, you can also measure a wide range of other data sets. Machine learning makes it easier than ever to put together data in real-time.
Tip -Use these numbers to tell a story that makes sense. If your sales are down, look at your efficiency to see if there is a link. Keep digging through these measures until you find a "why" for the problem you're trying to solve.
Organize Your Data
To make good business choices, you need to organize your data to make it easier to see. If you can't see all your important data in one place and understand how it all fits together, it's hard to know if the choices you're making are the best ones.
Tip -An executive dashboard is one way to keep track of your info. A customizable interface that is generally part of your universal reporting tool is an executive dashboard. This dashboard will show you the most important facts you need to reach your goals, whether they are strategic, tactical, analytical, or operational.
Perform Data Analysis
After you've put your data in order, you can start your data-driven research. This is when you'll get information from your data that you can use to help you make decisions.
Depending on your goals, you may want to combine the data from your executive dashboard with user research like case studies, surveys, or testimonials so that your results include the customer experience.
Does your team want to make its SEOtools better so that they can compete better with other options on the market? You can use the following data sets to figure out what changes need to be made:
- Competitors' performance data
- Current SEOsoftware performance data
- Current customer satisfaction data
- User research on a variety of SEO/marketing tools
Some of this information will come from your company, but you may also have to get some of it from outside sources. When you look at all of these data sets together, you can get a better idea of what's going on than if you just looked at each one on its own.
Tip -Give your whole team or company access to your analytics tools. Just like any other group project, data analysis works best when looked at from different points of view. Even though you might see one trend in the data, a teammate might see something totally different.
Draw Conclusions
As you look at your data and try to figure out what it all means, you'll probably start to come to some opinions. But your conclusions need their own part because it's important to explain what you see in the data so that you can share your findings with others.
- When coming to a conclusion, the most important things to ask yourself are:
- What am I seeing that I already knew about this data?
- What new information did I learn from this data?
- How can I use the information I've gained to meet my business goals?
Once you can answer these questions, you've done a good job of analyzing data and should be ready to use it to make business choices.
Tip- After analyzing the data, it makes sense to write down some SMART goals. Now that you've looked into the facts, you can use what you've learned to set goals that you can reach.
Successful Data-Driven Decision-Making Examples
Now that we have a better idea of what it means to make a data-driven choice and how important they are, we'll look at 5 great examples of data-driven decision-making.
According to a story on smartdatacollective.com, the search giant Google is one of the best examples of making decisions based on data. Startups are known for getting rid of hierarchies, and Google wanted to know if having bosses was really important.
Google's data scientists looked at performance reviews and employee polls from the managers' subordinates (qualitative data) to figure out the answer. The scientists put the information on a graph and found that most people thought managers were good. They took it a step further by dividing the data into the top and bottom quartiles and then running regressions.
The best and worst managers were very different in how well their teams worked, how happy their employees were, and how many employees they lost. What makes a good manager at Google? Good managers make Google more money and make workers happier.
Again, the analysts looked at the data from the "Great Manager Award" scores. Employees could suggest managers who did a great job. The workers had to give specific examples of what made the boss so good. To fill out the data set, managers from the top and bottom quartiles were also talked to.
Google's research found the top 8 things a good manager at Google does and the top 3 things that don't. They changed their training for managers to include the new information, kept the Great Manager Award, and started a twice-yearly feedback poll.
Walmart
In 2004, when Hurricane Frances was coming, Walmart used a similar method to get emergency goods ready. This was revealed by The New York Times. Managers wanted to know what kinds of goods they should stock up on before the storm. Their analysts looked through records of purchases made at other Walmart stores under similar circumstances.
They sorted through a terabyte of customer information to decide which goods to send to Florida (quantitative data). It turns out that Americans eat strawberry Pop-Tarts and drink beer when there is an environmental disaster.
During the storm, Walmart's analysts not only kept Floridians happy by giving them beer and Pop-Tarts, but they also made money by predicting how fast most of the goods would sell.
Southwest Airlines
Every business has a lot to gain from making decisions based on data, but the airline industry is one that is widely known to benefit from such insights. Executives at Southwest Airlines used focused customer data to learn more about which new services would be most popular with customers and make the most money.
By doing this, the airline found that it could give different groups of customers the best rates for their needs as well as a great customer experience (CX) by watching and analyzing their online actions and behaviors. Because of this focus on making choices based on data, Southwest Airlines' customer base and brand loyalty have grown steadily year after year.
Amazon
The industry talks a lot about Amazon as another case study of how data-driven decisions were made. The e-commerce giant makes correct product suggestions for users by combining information about what customers have bought in the past with techniques from behavioral analytics.
These suggestions are used at different points in the shopping process, from looking at products to checking out. This makes the process much more specific and easy for the user. For example, if a customer bought a cell phone, Amazon could suggest a case for the phone at checkout or in an email a few days later.
By keeping track of what each customer buys and how they like to buy it, Amazon can improve the shopping experience and increase sales and income at the same time.
Netflix
Netflix has more than 128 million active users and is the leader in the streaming service business because it uses data to keep customers. The company needed to find a way to improve the user experienceand make buyers want to stay on the platform because the industry was getting more and more competitive by the day.
They did this by carefully looking at metrics like watch time, date, location, the types of shows or movies a user usually watches, when a user stops or resumes content, and much more. With this knowledge, they are able to make a good recommendation algorithm that makes the viewer's viewing experience better.
After putting the program into place, the company looked at the results and found that almost 80% of users did what was suggested. Making it a good way to keep up with the competition and improve retention rates. A great example of making good decisions based on facts.
Challenges Of Data-Driven Decision Making
While Data-Driven Decision Making (DDDM) offers numerous benefits, it also comes with its fair share of challenges and obstacles. Here are some of the common challenges associated with implementing DDDM:
- Data Quality Issues - Poor-quality data can lead to inaccurate analyses and flawed decisions. Inaccuracies, inconsistencies, and incomplete data can undermine the trustworthiness of the insights derived from data.
- Data Privacy and Security - As organizations collect and use more data, concerns about data privacy and security become more significant. Complying with data protection regulations and ensuring the security of sensitive information is crucial.
- Data Integration Complexity - Integrating data from diverse sources can be complex. Different data formats, structures, and standards can pose challenges when trying to create a unified dataset for analysis.
- Lack of Data Governance - Insufficient data governance policies and practices can result in data silos, inconsistent data definitions, and conflicts over data ownership.
- Resource Constraints - Effective DDDM often requires significant investments in data infrastructure, tools, and skilled personnel. Smaller organizations may face resource constraints in building and maintaining these capabilities.
- Resistance to Change - Transitioning to a data-driven culture may encounter resistance from employees accustomed to traditional decision-making methods. Overcoming this resistance and promoting data literacy is crucial.
- Complexity of Data Analysis - Advanced data analysis techniques, such as machine learning and artificial intelligence, may require specialized expertise that organizations may lack.
- Overemphasis on Data - There's a risk of over-reliance on data, leading to analysis paralysis or ignoring valuable non-data-driven insights, such as expert opinions or customer feedback.
- Ethical Concerns - DDDM can raise ethical questions, especially when using data for profiling, personalization, or decision-making that affects individuals. Ensuring fairness and avoiding discrimination is essential.
- Data Bias - Data used for analysis may contain inherent biases, which can lead to biased decision-making if not properly addressed. This bias can be due to historical patterns or data collection methods.
- Data Interpretation Challenges - Interpreting data correctly and drawing meaningful insights can be challenging. Misinterpretation or misapplication of data can lead to misguided decisions.
- Data Volume and Velocity - Dealing with the sheer volume and speed at which data is generated can be overwhelming. Real-time data and big data present unique challenges in terms of storage, processing, and analysis.
- Legal and Regulatory Compliance - Organizations must navigate complex legal and regulatory frameworks when collecting, storing, and using data, which can be time-consuming and resource-intensive.
- Measuring Impact - Determining the causal relationship between data-driven decisions and outcomes can be challenging. Attribution of success or failure to specific decisions may not always be straightforward.
Data-Driven Decision Making FAQs
What Are The Benefits Of Using Data-driven Decision Making In Marketing?
Using DDDM in marketing can lead to improved targeting, higher ROI, better customer engagement, and more effective campaigns. It allows marketers to make decisions based on data insights rather than guesswork.
How Can Small Businesses Implement Data-driven Decision Making On A Budget?
Small businesses can implement DDDM by focusing on essential data collection, utilizing free or low-cost analytics tools, and leveraging cloud-based solutions. Prioritizing data quality and investing in employee training can also be cost-effective strategies.
What Are Some Common Mistakes To Avoid In Data-driven Decision Making?
Common mistakes include relying on biased data, misinterpreting data, neglecting ethical considerations, and overcomplicating analysis. It's essential to address these pitfalls to ensure the accuracy and effectiveness of DDDM.
Can Data-driven Decision Making Be Applied In Healthcare?
Yes, DDDM is highly applicable in healthcare. It can help improve patient care, optimize hospital operations, identify disease trends, and enhance clinical outcomes by leveraging patient data, medical records, and research findings.
What Is The Role Of Artificial Intelligence (AI) In Data-driven Decision Making?
AI plays a significant role in DDDM by automating data analysis, predictive modeling, and decision recommendations. Machine learning algorithms can uncover insights from vast datasets, making AI a valuable tool for informed decision-making.
Conclusion
Data-driven decision-making is not a fleeting trend but a strategic approach that has become an integral part of our modern decision-making landscape. As we continue to generate and accumulate vast amounts of data, the importance of harnessing this information for informed choices will only grow. Whether in business, healthcare, government, or personal decision-making, DDDM empowers us to make better choices, mitigate risks, and seize opportunities.
It's a tool for those who seek precision, efficiency, and a competitive edge in a world where data is king. Embracing DDDM is not just a choice; it's a necessity in a data-driven future where success is guided by insights, not instincts.